Jun 27, 2024 · 9 min read
Exploring the ‘Digital Age of Biology’ With CZI’s Science Technology Team
Teams across the organization and its scientific institutes came together to discuss how advances in AI can uncover fundamental properties of human biology.
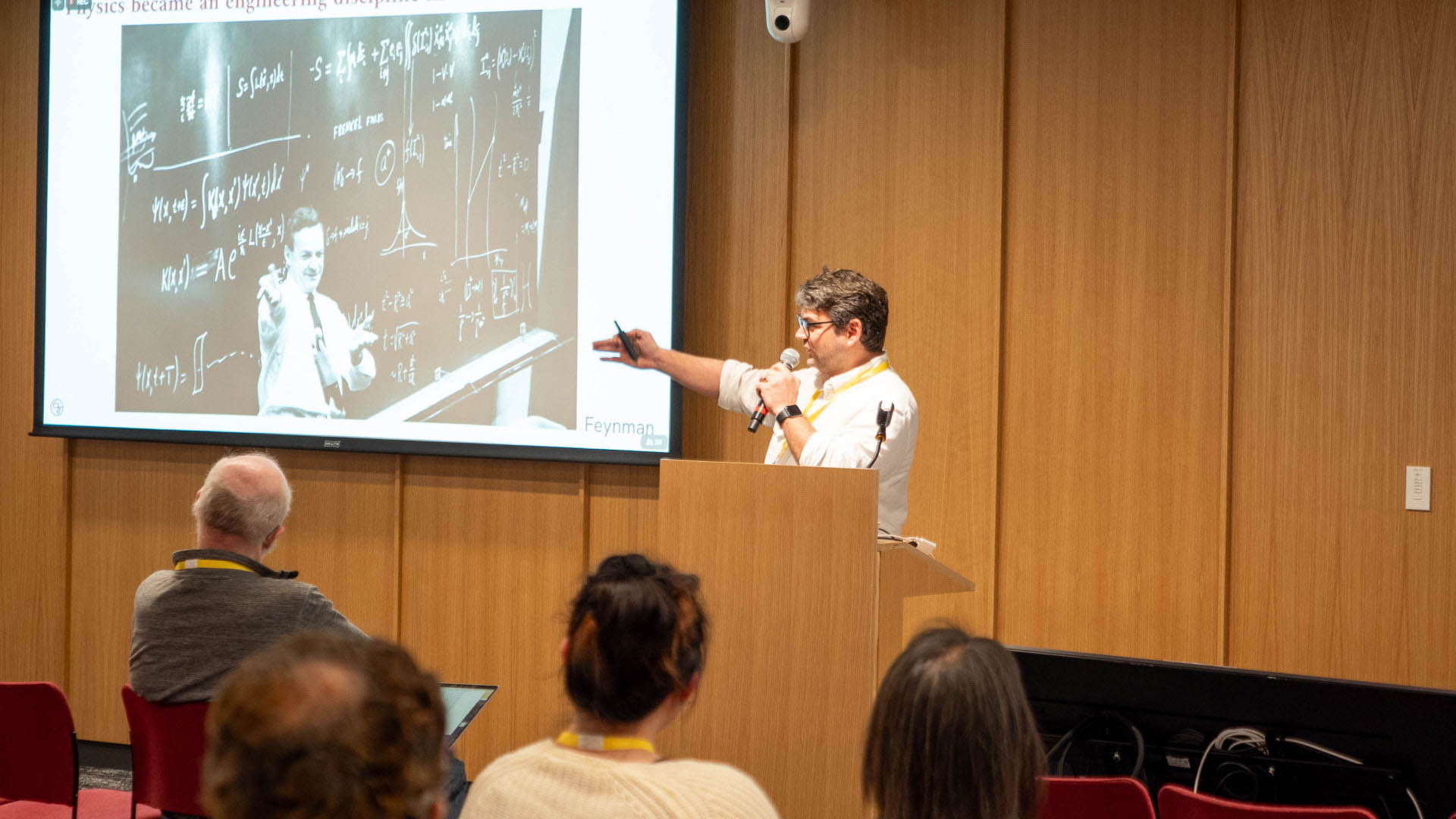
Seventy-five years ago, the mathematician and computer scientist Alan Turing posed a simple but powerful question that changed the course of technology: Are machines capable of thought?
Since then, artificial intelligence has advanced at an extraordinary pace, and today, it’s opening the door to the “digital age” of biology.
From leveraging machine learning to help visualize the location and interactions of proteins within live cells to training a deep-learning model that can predict the impact of gene perturbations in cell types or genes, the application of AI methodologies to make sense of and draw insights from massive amounts of scientific data is ushering in a new level of insights into human health and disease.
This theme was the main focus of CZI’s recent science technology convening, which brought together computational biologists, engineers, data scientists, product designers, and leaders from across the organization and our family of scientific institutes to explore the frontiers of AI for biomedical research. CZIers and our collaborators led sessions on topics ranging from how machine learning is expediting the annotation of tomograms from cryo-electron tomography experiments to building customized ultraviolet microscopes to detect and diagnose malaria in low-resourced settings.
Industry AI experts — including Boris Power, head of applied research at OpenAI, and Bryan Catanzaro, vice president of applied deep learning research at NVIDIA — also led talks about the promise of training AI models to expand the scientific community’s foundational understanding of human biology.
Three main themes surfaced after two days of enriching discussions:
- Basic science goes hand-in-hand with AI.
- Training models on multimodal datasets holds enormous promise for the future of research.
- Shifting from focused to general-purpose generative models for scientific research will open the door to many new biomedical discoveries.
Take a closer look at these takeaways below.
Basic Science Goes Hand-in-Hand With AI 🤝
“Biologists are going to have very strong simulations enabled by virtual cell models — in a way that’s not possible today,” said Steve Quake, CZI’s head of science, during the opening remarks. His point emphasized how AI will fundamentally change and accelerate the way scientists do research in the coming years.
For example, the virtual cell models CZI is building will be able to predict the response of immune cells to different genetic mutations faster and in more robust combinations than current methods without the need to collect costly and invasive physical samples from patients. It’s like having a combination lock for human biology — once you have the code, it will open up a host of new information about what happens when cells become diseased and what it takes for them to become healthy again.
Marinka Zitnik, assistant professor of biomedical informatics at Harvard Medical School and associate faculty at the Kempner Institute for the Study of Natural and Artificial Intelligence, led a session that further highlighted AI’s role in transforming scientific research in the context of her day-to-day work. Zitnik, a CZI collaborator and Science in Society grant partner, highlighted how machine learning algorithms are being used to augment research and provide new insights at different time and spatial scales.
One example is SHEPHERD, a deep learning approach built by Zitnik’s team that can provide individualized diagnoses of rare genetic diseases. Given the limited data on rare diseases, the model is pre-trained on known associations between variants, genes and phenotypes from patient-agnostic data. The model is then trained on simulated patient data before being fine-tuned in the real world, potentially speeding up diagnoses and improving patient outcomes.
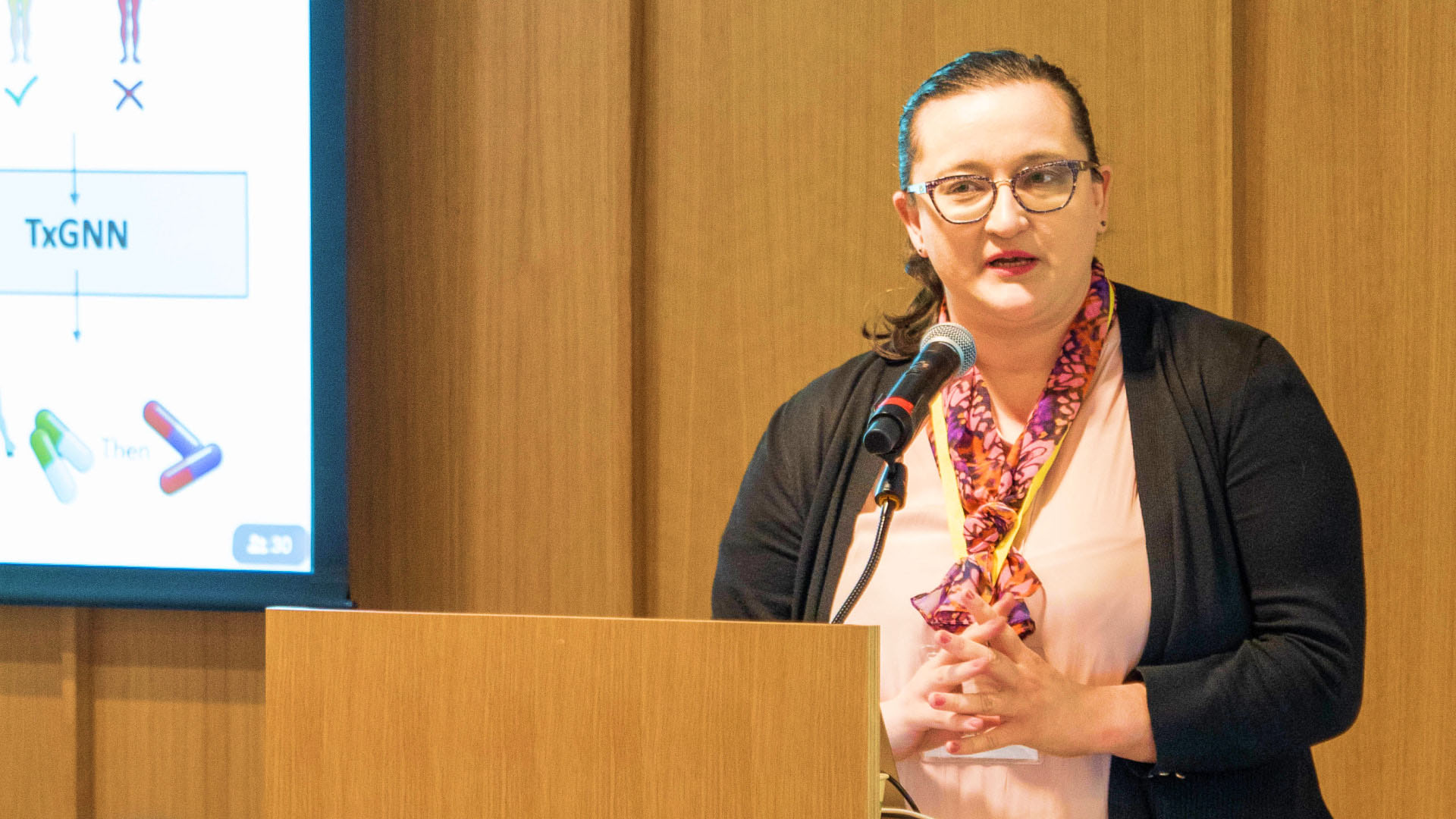
When evaluated across 12 sites throughout the United States, SHEPHERD was able to nominate disease-causing genes for 75% of patients from a cohort affiliated with the Undiagnosed Diseases Network. The model also narrowed down the top five possible genes responsible for those diseases among the tens of thousands of genes prioritized by the model. By providing a broad characterization of novel diseases — helping researchers identify genes harboring mutations that can lead to disease, and connecting patients with similar genetic and phenotypic features for potential clinical follow-ups — SHEPHERD is fundamentally changing the way researchers like Zitnik study and develop potential therapeutic targets for rare diseases. This can shorten the time for diagnosis and improve outcomes for patients.
A Multimodal Data Oasis 🌊
Over the last decade, scientists, academic research labs and philanthropic organizations like CZI have been collecting, aggregating and curating enormous amounts of detailed, high-resolution biological information about the trillions of cells within the human body. These datasets are sequence- or image-based — two complementary modalities that are fundamental to advancing biomedical research.
Manu Leonetti, director of systems biology at the Chan Zuckerberg Biohub San Francisco (CZ Biohub SF), and James Zou, associate professor of biomedical data science at Stanford University, led discussions about the opportunities with training AI on multimodal datasets. Leonetti, a cell biologist, described imaging as one of the “foundational modalities” for biology, allowing scientists to explore advanced techniques like transcriptomics under a microscope.
“Imaging has the power of being able to give us extremely dense multimodal profiles of cells,” said Leonetti. “We can ask questions across scales while following cells in the context of their native environment, whether looking at cells in a dish, or tissues, or even at the scale of an entire organism.”
New developments in deep learning are fueling the power of imaging. At the CZ Biohub SF, for example, Leonetti and his colleague Loic Royer are developing new algorithms to extract functional information from biological images. Royer’s imaging AI team has also trained a de-noising algorithm called Aydin that dramatically increases the usability of microscopy images and tools that can recognize and quantify biological objects from complex images to accelerate analysis.
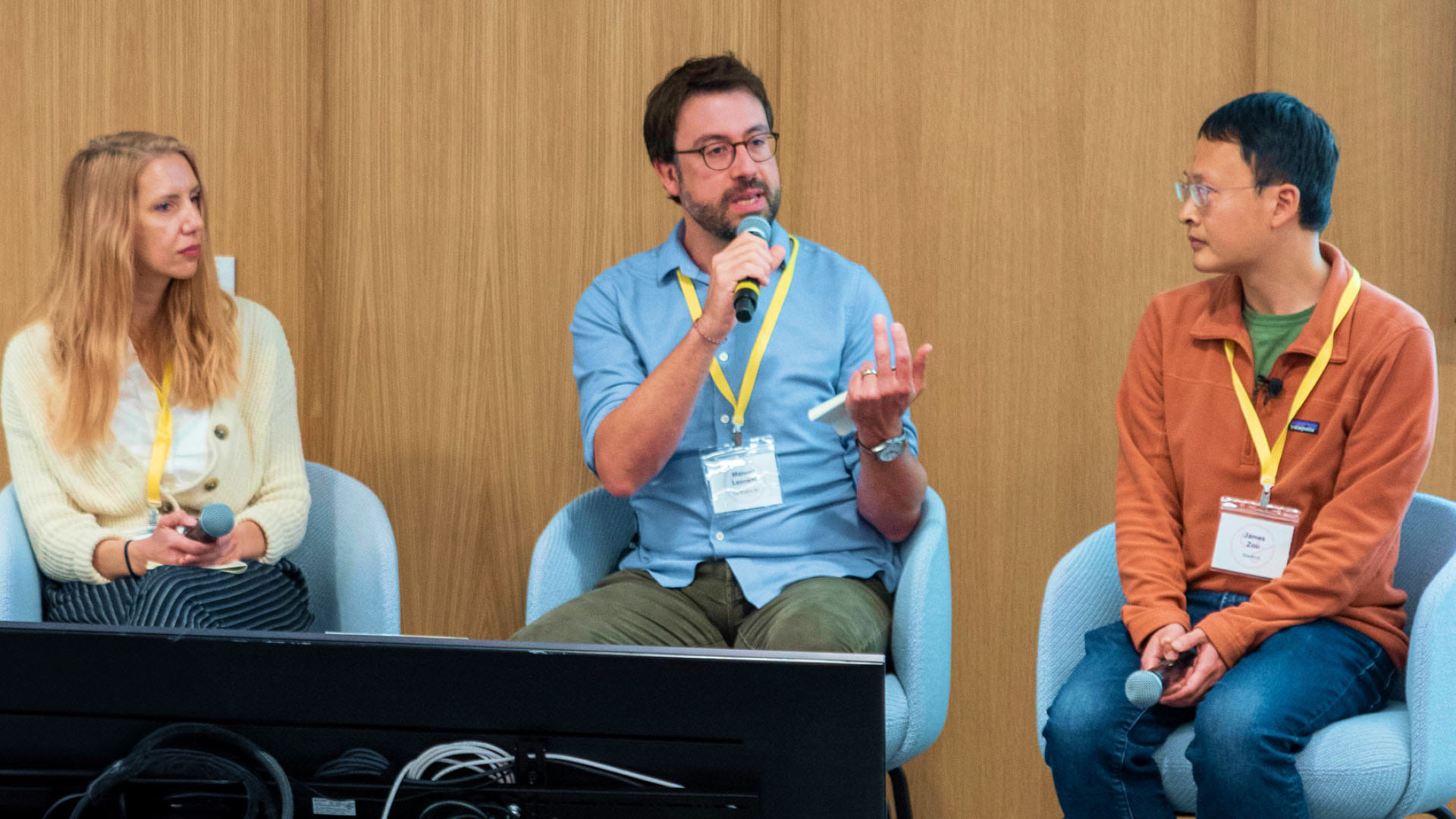
Zou also shared examples of how generative AI is transforming biomedicine, including a case study showing how models can help identify and synthesize molecules to guide the development of antibiotics.
“Generative AI can really help us expand the search space,” said Zou. “If we can use the help of AI to explore small molecules that we have not seen before in nature and are likely good drug candidates, that can likely be transformative for drug discovery.”
The deep learning method Zou cited pinpointed a candidate molecule that could fight against various pathogens, including antibiotic-resistant bacteria. This breakthrough comes at an especially critical time, given the rise in antibiotic-resistant pathogens globally.
On the topic of modalities, Zou shared his perspective on why language can be a unifying framework for integrating vast amounts of biological information.
“The reason why I’m particularly excited about language is the knowledge that’s summarized in written text,” he said. “There’s a lot more information captured in language beyond what’s shared in numerical data.”
To illustrate this point, Zou zeroed in on recent advances in protein modeling like ESMFold and AlphaFold, which draw correlation patterns from sequences. While these models are powerful tools for making predictions about protein structure, they aren’t trained on existing literature about the role and function of different proteins.
However, Zou also said that fine-tuning these protein language models with information from existing literature — decades of knowledge summarized in papers — leads to a notable boost in the capability of these models.
A ‘General-Purpose Model’ To Power Basic Science 💪
Today, most of the field’s AI models are designed for applications in specific research areas, whether in the context of identifying genetic mutations that can lead to rare diseases or identifying new molecules that can overpower antibiotic-resistant pathogens.
But in the future, CZI’s goal is to build and train a “general-purpose model” or virtual cells that can transfer information across datasets and conditions, serve multiple queries concurrently, and unify data from different modalities.
Explore more: How AI Can Uncover the Laws of Biology
Theofanis Karaletsos, CZI’s head of AI for science, provided attendees with a closer look at our vision for building a general-purpose model that can serve as a foundational resource for biomedical research. Karaletsos started his talk by highlighting the extraordinary amount of biological information generated over the last decade, which is breaking Moore’s Law.
By bridging the gap between these datasets and advances in AI, “we get to the heart of where we want to be as machine learners,” said Karaletsos. “We want to simulate a generative process such that in some coarse-grain level of casualty — even if it doesn’t get things exactly right at a fine level — but at some level, we’ll have useful models that will allow us to ask questions about the data and query them in interesting ways for counterfactuals.”
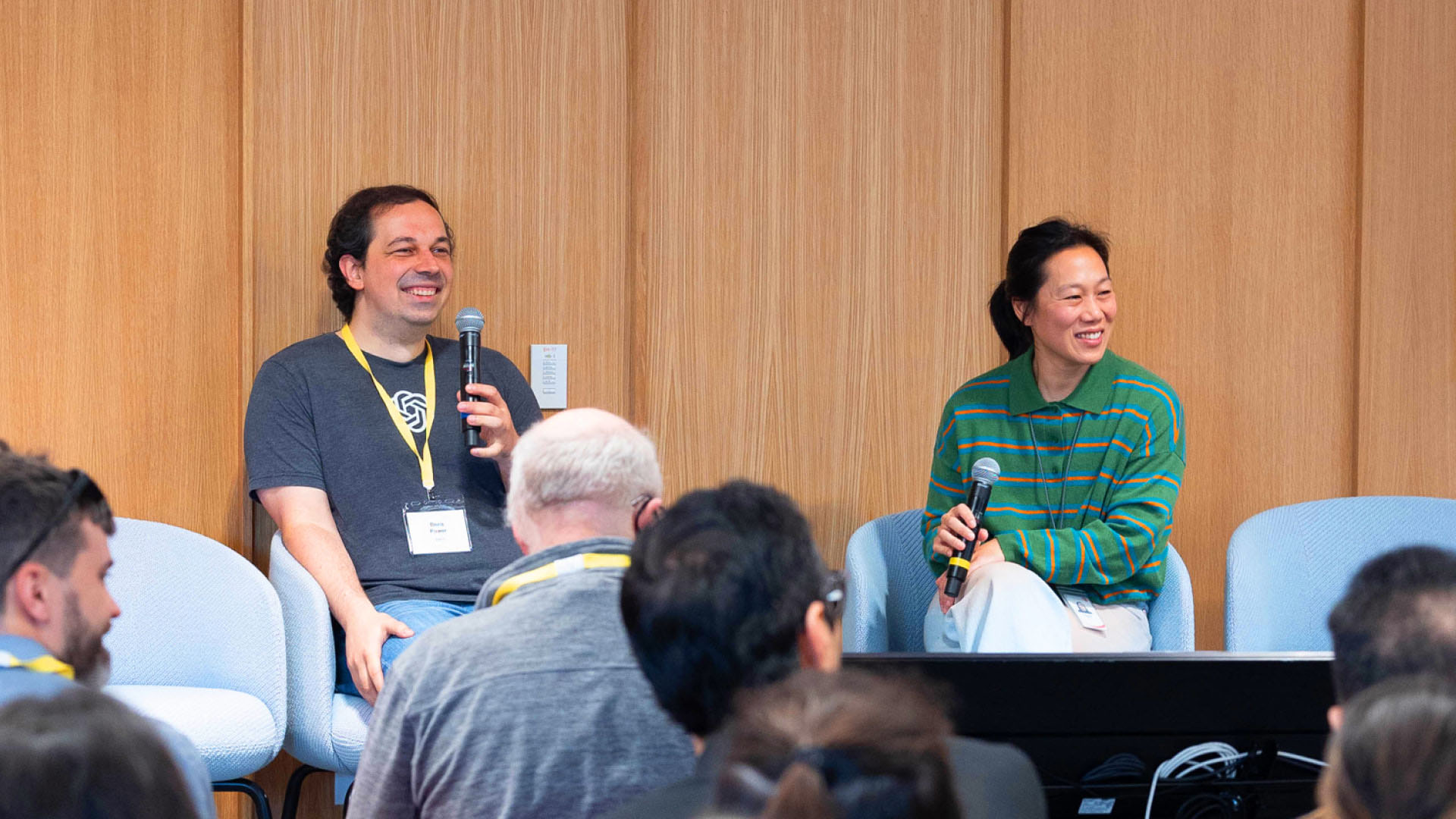
To bring these virtual cell models online, the early days of CZI’s AI strategy will focus on training models and making these models and the datasets used for training and validation available to the community, which will require deep cross-functional collaboration with our teams, AI/machine learning experts, and biologists using these models.
Ultimately, this approach will pave the way for an open, accessible digital platform for biology, which will house next-generation models and systems trained on expansive multimodal datasets. Scientists will be able to access these models via APIs and visualizations to pose complex questions and test theories about the fundamental mechanisms of human biology faster and more accurately than traditional experimentation methods and existing, more specialized generative AI models.
“Over time, we want this to handle basic biology tasks,” Karaletsos concluded. “We hope it’ll be useful for disease and ultimately for cellular engineering because we want to understand cells in a generative way.”
Learn more about CZI’s AI strategy for science and our vision to build predictive models of cells and cell systems.