Efficient Data Structures for Single-Cell Data Integration
Project Summary
Single-cell experiments are rapidly increasing in scope, generating formidable computational challenges for integrative analysis. This project democratizes atlas-scale integration of single-cell data with a new sparse matrix format that requires 1/10th the space of current standards without compromising performance. A new library for additive non-negative matrix factorization (NMF) facilitates the analysis of massive datasets, yields interpretable joint models of coordinated gene activity programs, and operates an order of magnitude faster than current standards.
NMF relaxes assumptions of orthogonality to instead superimpose additive biological signals, revealing context-dependent gene activity programs and cellular identities. Co-regulation of genes or elements can be assessed by domain experts such as physicians or biologists without extensive training. Performant out-of-core normalization, dimension reduction, clustering, and visualization schemes benefit both analysts and domain experts, reducing computational burdens and allowing comparison of multiple approaches to suit experimental or exploratory aims. This approach operates out-of-core on arbitrary data slices, thus avoiding the need for distributed processing in most applications. As proof of principle, these novel methods can learn joint models of coordinated gene activity across organisms, disease contexts, and tens of millions of cells, all at previously unattainable resolution. These tools further lower barriers to understanding rare diseases, orphan genes, and novel perturbations, and will advance the adoption of single-cell atlases across and outside traditional academic silos.
Investigators
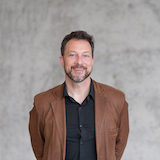