Semantically-Coherent & Statistically-Comparable Representation of Reference Cell Types
Project Goal
To develop a scalable approach for semantically-coherent and statistically-comparable cell type definitions, using data from emerging high-throughput, high-content and high-resolution technologies.
Results & Resources
During this project, the Scheuermann lab devised a strategy for defining cell types based on identifying the minimum set of necessary and sufficient marker gene or protein expression patterns. To this end, they developed a machine learning algorithm, NS-Forest, which takes as input an scRNA-seq gene expression matrix and produces the minimum set of marker genes needed. A step-by-step protocol for NS-Forest is openly available. Additionally, they developed a new cell type cluster comparison method, FR-Match, which is a novel application of the Friedman-Rafsky test, a non-parametric statistical test for multivariate data comparison.
Investigators
Lead Investigator
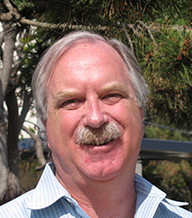