Semi-Supervised Generative Autoencoder Models for Single-Cell Data
Focus
Latent Spaces
Project Goal
To develop deep neural network (autoencoder) models for single-cell data and testing their application to generate a suitable data representation for downstream analysis of cell phenotypes, such as metabolic activity prediction.
Results & Resources
The Heinaniemi lab developed four semi-supervised network models that were benchmark against three published methods using CITE-seq data. All data and models can be found on their github repository, and the models are further described in their preprint.
Investigators
Lead Investigator
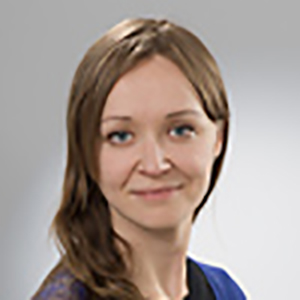
Merja Heinaniemi