Fast, Interactive Instance Segmentation with Mutex Watershed
Project Summary
In bioimage analysis pipelines, instance segmentation is the key step that extracts objects of biological interest from potentially very large image volumes. This project aims to develop a napari plugin for interactive segmentation based on the Mutex Watershed algorithm. This efficient algorithm can segment objects in 2D and 3D images, based on predictions from a Convolutional Neural Network (CNN), which can be further enhanced by light user annotations for splitting or merging objects. High accuracy of this step is paramount for the validity of downstream analysis. Several deep learning based tools exist for this task and provide pre-trained models for common modalities. However, in practice these (fixed) pretrained models do not yield high enough quality results due to the diversity of imaging modalities and biological specimens.
A napari plugin offers the ideal platform to make this method available to the wider bioimaging community. The plugin will support fast and interactive segmentation, provided along with pre-trained networks for some of the most challenging microscopy segmentation problems. It will significantly reduce the manual effort needed for instance segmentation in new and existing data.
Investigators
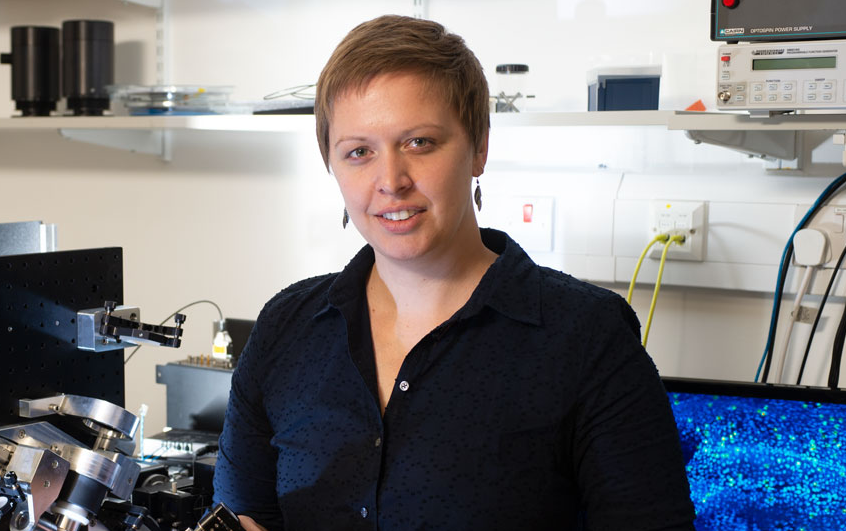
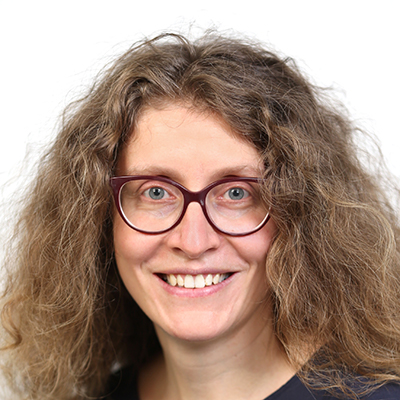