Diffusion and Deep-Learning Based Methods for Exploratory Data Analysis
Focus
Latent Spaces
Project Goal
To learn data manifolds via diffusion and deep learning in order to denoise, extract structures such as clusters or trajectories, and to visualize data.
Results & Resources
In this project, the Krishnaswamy lab developed SAUCIE, a deep learning toolbox for biomedical data exploration that provides scalable clustering, data imputation, visualization and batch correction. This furthered their work on multi-view diffusion geometry to yield a new data fusion approach, harmonic alignment. Additionally, they developed a method, called diffusion condensation, that slowly condenses data towards near neighbors in a continuously hierarchical tree. To find gene interactions they developed a dynamics modeling neural network that follows trajectories, DyMON.
Investigators
Lead Investigator
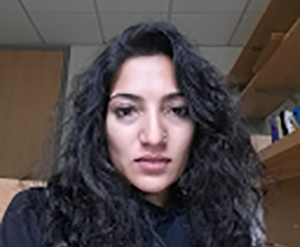
Smita Krinshnaswamy