Genome-Wide Hypothesis Generation for Single-Cell Expression via Latent Spaces
Focus
Latent Spaces
Project Goal
To build enabling software and datasets for latent-space transformations of Human Cell Atlas data.
Results & Resources
The Greene lab developed a machine learning approach, MultiPLIER, that extracts latent variables from large collections of public data. Using this approach, they found that in the context of rare disease, it is possible to identify cell types and processes that are misregulated in small, existing bulk datasets.
Investigators
Lead Investigator
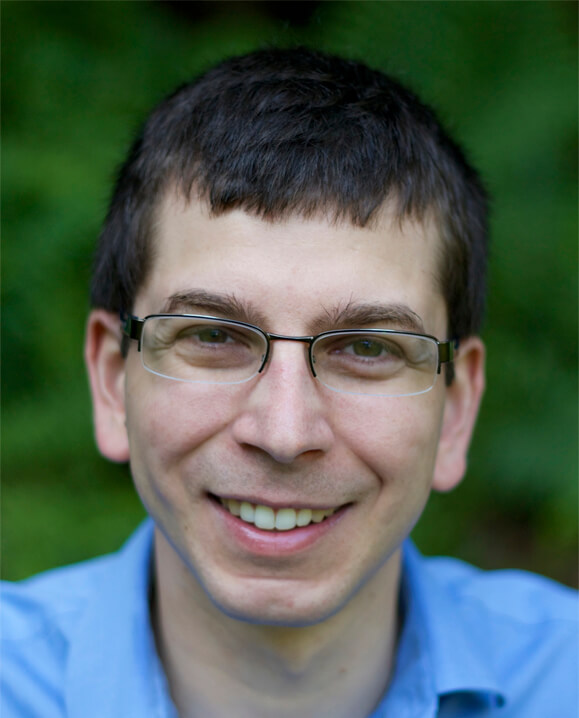
Casey Greene