Machine Learning Methods for Fully Exploiting Heterogeneous, Multimodal Data
Focus
Multiomics
Project Goal
To develop novel statistical methods enabling the fusion of multiple data types collected via different experimental technologies.
Results & Resources
This group published four papers on improving computational scalability, multiple hypothesis testing, and measurement linkage. They also developed a new computational method, BARcode DEmixing through Non-negative Spatial Regression (Bardensr), to meet the demand for a high spatial density of transcription required for quality imaging resolution. Together, these tools provide scalability and improved integration of multimodal data.
Investigators
Lead Investigator
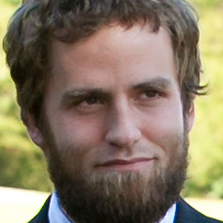
Liam Paninski