Nonparametric Manifold Learning for Imputation and Alignment in Single-Cell RNA-sequencing Data
Focus
Manifolds
Project Goal
To develop a generative model of single-cell RNA-seq data that allows normalization, imputation, and batch correction for the purpose of identifying QTLs across many individuals.
Results & Resources
This project developed tGPLVM, a software used to estimate low-dimensional embeddings for filtered and normalized single-cell data. This group also developed sstGPLVM, a model that projects data onto a mixture of fixed and latent dimensions, and learns a unified low-dimensional embedding for multiple single-cell experiments with minimal assumptions. Combined, these tools help represent very complex datasets and improve the field’s ability to perform multi-model analysis that spans studies and measurement techniques.
Investigators
Lead Investigator
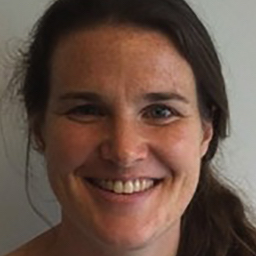
Barbara Engelhardt