Quantization and Compressive Learning Methods for Omics Data
Focus
Compression
Project Goal
To develop new omics data quantization and lossless compression algorithms and accompanying machine learning methods that are robust to quantization errors.
Results & Resources
The Ochoa lab, in collaboration with Mikel Hernaez, developed a number of genomic data compressors:
- SPRING, for FASTQ files, is able to reduce the size of compressed raw genomic data by a factor of three.
- ALICO, for SAM/BAM files, achieves 20% improvement over other tools while maintaining similar speeds.
- GPress, for gene expression files, achieves a 98% reduction in file size while maintaining the ability to retrieve all annotations for a given identifier or a range of coordinates.
- MassComp is a compressor for mass spectrometry data from proteomics and metabolomics studies.
- DeepZip, a general compressor for any type of data, is based on neural networks and when applied to genomic data, achieves a comparable performance to that of specialized compressors.
Investigators
Lead Investigator
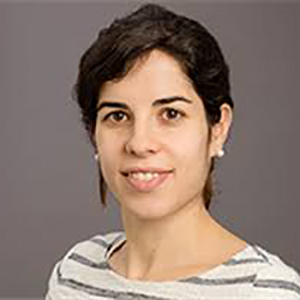
Idoia Ochoa