Deep and Standardized Single-Cell Annotations with CITE-seq
Project Summary
Cell type annotation in single-cell analysis is a common problem required for biological interpretation and downstream statistical analyses. While much progress has been made in this area, current state-of-the-art techniques still suffer from major limitations including lack of ground truth, reliance on limited reference datasets, and lack of standard annotations. As such, there is still no preferred approach, and current best practice relies on a combination of methods, most often followed by manual annotation/curation. This introduces bias, since these strategies will tend to focus on cell subpopulations deemed important a priori by the investigator. Non-standard labels also make it difficult to compare results and integrate datasets across studies. With CITE-seq (and related technologies), protein expression of cells can be directly measured in addition to RNA expression (and possibly other modality, e.g. epigenetic), facilitating robust and deep cell type annotation. Despite their great potential, protein expression data are often analyzed using tools developed for single-cell RNA-seq even though the characteristics of the data are substantially different.
This project will develop tools specifically tailored to protein data, including normalization and annotation, and leverage public databases to create a corpus of well-annotated single-cell data with deep and standardized annotations. The team will then use these annotated data to develop a pre-trained machine learning model that can be applied to scRNAseq data for predicting derived annotations even in the absence of protein measurements, facilitating biological interpretation and cross-study comparisons of any given dataset at great depth with standardized labels.
Investigators
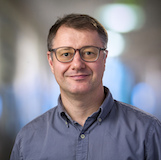
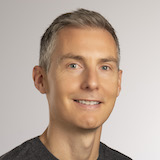