Multi-omics Integration with Batch-adversarial Neural Networks
Project Summary
A wealth of single-cell protocols makes it possible to characterize different molecular layers at unprecedented resolution. Connecting multiple (single or paired) readouts holds the promise to move from profiling cellular states to an understanding of the underlying molecular (e.g. gene regulatory) networks. Yet integrating multimodal single-cell data to find cell-to-cell correspondences remains a challenge. Data integration needs to happen at a meaningful level of abstraction; considering the inherent discrepancies between modalities is needed to strike a balance between biological discovery and noise removal. Given its novelty, there is limited availability and even less insight into computational methods that address this topic in a principled manner.
This team recently published BAVARIA, a flexible approach that allows for competitive and effective simultaneous batch integration and dimensionality reduction of replicate scATAC-data, even across different experimental platforms. The team has now extended this framework to multimodal inputs that use a shared narrow latent space.
This team hopes to turn this prototype, LIAM, into a comprehensive tool with capabilities to have flexible inclusion of new modalities and semi-paired training strategies via combination of single and paired multi-ome datasets. The project also aims to automatically determine batch integration strength across a range of scenarios, from technical replicates to environmental or genetic perturbations. This project will accompany this with a meta-assessment of the suitability of benchmarking criteria to truly reflect the desired biological properties.
Investigators
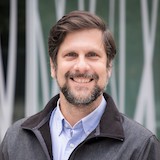