Development of Denoising Methods
Project Summary
Temporal resolution in any optical imaging system is limited by the amount of photons one can meaningfully utilize to form an image before destroying or otherwise negatively impacting the sample. To reduce this effect, exposure to light should be kept at a minimum. The observation of biological processes in healthy or diseased states is often not straightforward under reduced light conditions. Therefore, the role of image denoising to facilitate or even enable quantitative image analysis cannot be overstated.
This team will develop a plugin for napari that will allow users to train and/or apply previously trained self-supervised denoising models to the currently open 2D image, 3D volume, or time series. This group has supported the development of a powerful and extensive toolbox for self-supervised and unsupervised denoising, including Noise2Void (N2V) and its variants PN2V and PPN2V, DivNoising, or DecoNoising, which additionally can predict a denoising and deconvolved output. By bundling these denoising and image restoration methods in one convenient and easy-to-use, napari-based toolbox, this project will lay the groundwork for further growth of new methods and the development of additional variations.
Investigators
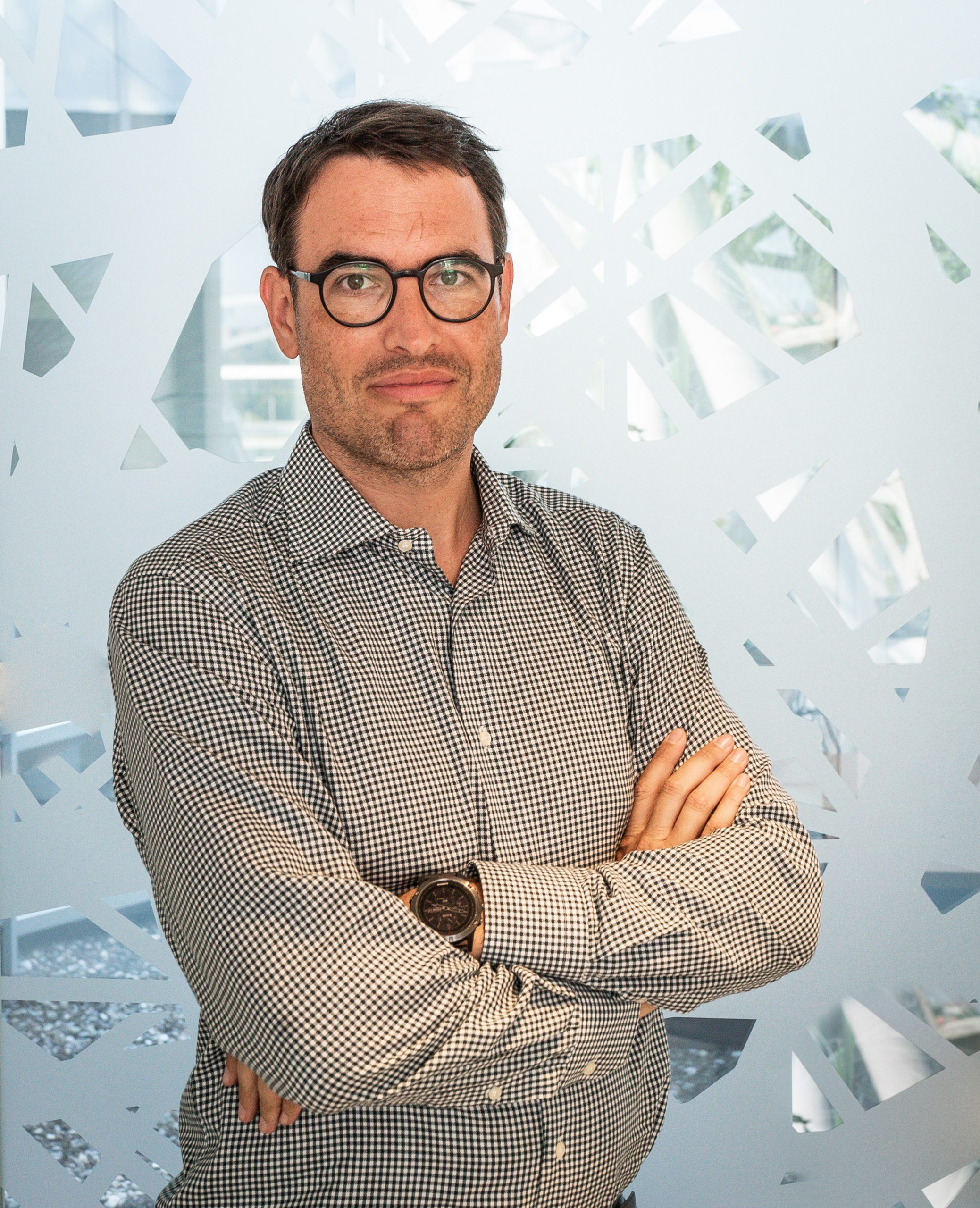
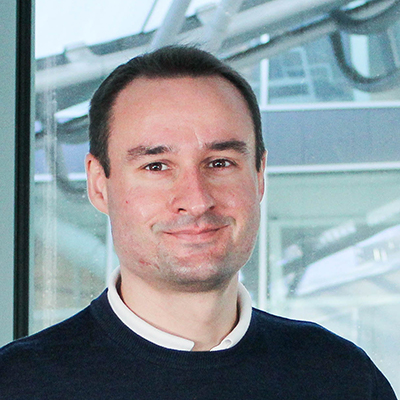
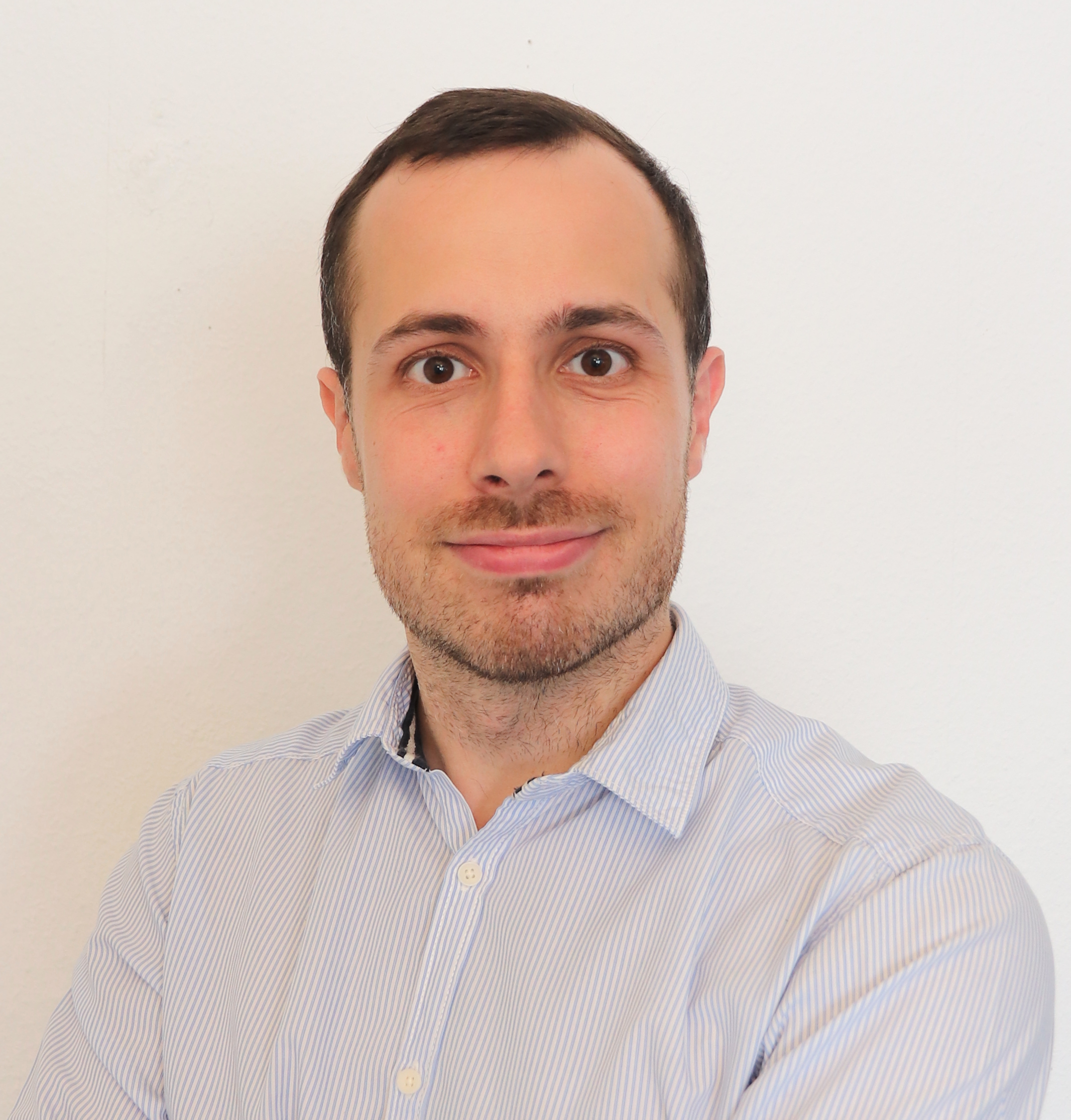