Statistical Methods to Investigate Phenotypic Variation
Project Summary
Gene co-expression network analysis is a key inference tool for detecting latent relationships invisible to standard workflows of clustering and differential expression analysis. Such a network approach was instrumental in bulk RNA-seq analysis to link genes with biological processes and discover candidate disease genes. While estimation of gene-gene correlations to construct co-expression networks is well-established for bulk RNA-seq, single-cell measurements of expression pose unique challenges due to technical limitations and noise levels inherent to the technology. In addition, emerging scRNA-seq datasets in population-based settings across multiple individuals and time points/perturbation systems are creating an unprecedented opportunity to quantify expression variation across individuals at the network level. These dynamic, multi-subject scRNA-seq datasets warrant novel methods to construct personalized dynamic gene networks and infer modules and network properties that drive phenotypic variation.
To address these challenges, this project will develop a measurement error model to estimate gene-gene correlations from scRNA-seq data and detect correlations that are otherwise hidden by technical limitations. The team will also innovate a global regularized spectral clustering method that takes in co-expression quantifications of genes at the subject and time/perturbation levels and infers dynamic gene modules across the subject and the time/perturbation domains. Both aims will deliver foundational tools applicable to a wide range of scRNA-seq datasets and are uniquely positioned for analyzing population-level scRNA-seq data. Leveraging team expertise to perform perturbation experiments in the hematopoietic system, the group will validate and benchmark these methods in this system where large collections of scRNA-seq, along with orthogonal single-cell data modalities, exist.
Investigators
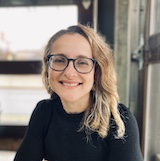
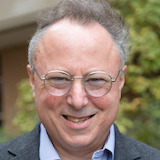