Dec 9, 2021 · 47 min read
Listen: Discussing the Future of Science Technology with Neuroscientist Edward Boyden
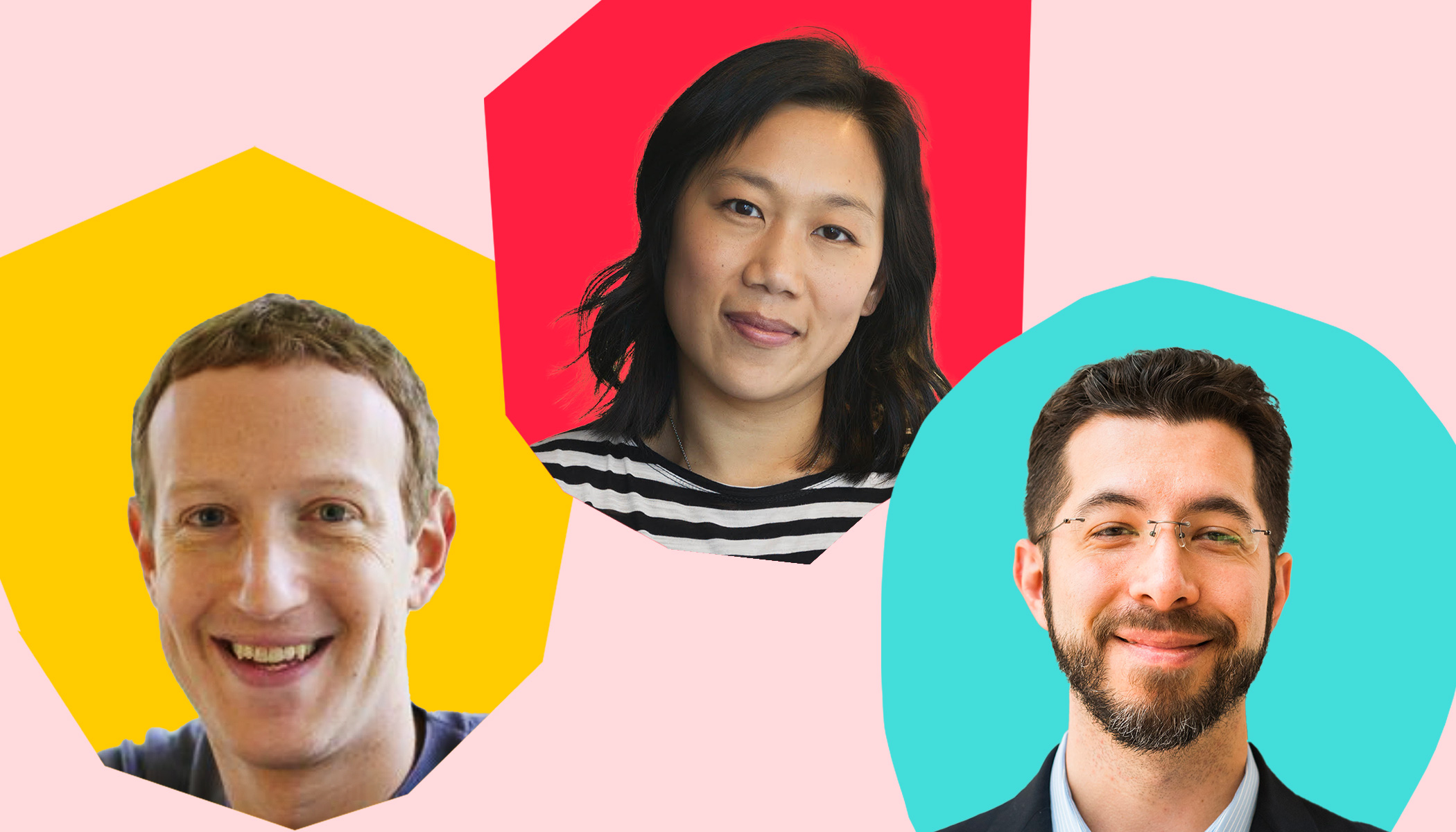
Dr. Priscilla Chan and Mark Zuckerberg, co-founders and co-CEOs of the Chan Zuckerberg Initiative, joined neuroscientist and professor Edward Boyden, Co-Director of the MIT Center for Neurobiological Engineering, for a Facebook Live Audio Room to discuss the future of biomedical science from the perspective of imaging science, technology development, and artificial intelligence. They also talked about what it will mean to observe and measure human biology in new ways, across spatial scales and in real time. Listen to the full talk, or read the full conversation transcript below.
Mark: Hey everyone. Thanks for joining today. I’m here for a conversation with Priscilla and Ed Boyden, who is an acclaimed neuroscientist, to talk about some of the future of biomedical science. And Ed has a distinguished career in developing imaging technologies, as well as a lot of other biological advances. So it’s really a pleasure to be able to spend some time talking to him about this. And we’d love to go through some of the new science programs that the Chan Zuckerberg Initiative announced yesterday and what’s most exciting for the future. So, Priscilla, do you want to kick us off with a more formal introduction for Ed and all of the awesome things that he’s done?
Priscilla: Sure, I’d love to. I mean, it’s hard to encapsulate Ed’s energy and the feeling of being in Ed’s lab, but I will try. There’s many accolades that I can go through. Ed is the Eva Tan professor in Neurotechnology in MIT. He’s a Howard Hughes Medical Institute investigator, that is a big deal. He’s a member of the McGovern Institute for Brain Research. He’s the co-director of the MIT Center for Neurobiological Engineering. He won the 2016 Breakthrough Prize in Life Sciences for his work in making transformative advances towards understanding living systems and extending human life. Every day in his lab, Ed is developing new tools and technologies that make it possible for us to see complex medical systems with nanoscale precision, teeny, teeny tiny connections.
And to enable us to understand and both control neurodynamics, which means what’s happening in your brain, with millisecond precision. One of those really cool technologies is called expansion microscopy, which allows us to expand and see biological systems in the nanoscale precision. It’s really cool, diapers are involved if he’s going to tell us about it. That’s right, baby diapers. He’s built optogenetic tools, which allow us to both see, activate and silence brain activity using light in other really cool noninvasive ways of stimulating the brain. Using these really wild, innovative, creative techniques, he’s been able to map molecules and the wiring of the brain to help us understand how the brain works and to repair the brain when it’s not working.
All these discoveries have now translated to really cool clinical trials on how we can impact and improve lives for people, including issues around blindness and Alzheimer’s. So that’s a lot, but you need to hear it from Ed. Thank you for joining us Ed in this conversation.
Ed: It’s great to be here with you Priscilla and Mark.
Mark: So I thought maybe before we jump in to the conversation, it might be helpful to give a little bit of a recap on the big CZI announcements from yesterday around the direction that we’re heading in for the next five years. So I’ll try to give a quick summary on this. Yesterday was the five-year anniversary of the Chan Zuckerberg Initiative Science Program. We launched it five years ago with a long-term goal to help scientists cure, prevent, or be able to manage all diseases within our children’s lifetime. And this is a goal that we think is possible, but it’s a long-term, multi-decade goal and journey.
We spent the first five years trying to work on a number of important scientific areas, but very focused on figuring out what types of work that we could do to bring science and technology, and enable new types of collaborations to accelerate scientific progress as much as possible. And after five years, I think we have more of a sense of which of those models and paradigms really worked and clicked and what to double down on going forward. So yesterday we announced our vision and strategy for the next five to 10 years of the Chan Zuckerberg Initiative Science Program, which is going to be about trying to build tools to observe and measure a lot of different aspects of human biology in action in living people.
Which poses a number of interesting challenges that we’ll talk about with Ed today. And a lot of the part of the strategy comes from, anytime that I’ve tried to solve a complex problem I’ve always found that it really makes sense to dedicate some time upfront to building tools to be able to measure the progress that you’re making, or measure and understand the problem directly. As an engineer, it’s hard to imagine trying to debug code without being able to step through it, but that’s where we are on biology today. Because today, the current state of microscopy and imaging is you can’t really see cells directly interact in living organisms or in people, at least. So that’s just one example of a type of tool, high-resolution in vivo technology for imaging that we set up an institute or we’re working on that for advanced imaging.
And that’s one of the three things that we announced that we’re doing yesterday. The other two, just to continue with a quick summary of this, is one of the more successful experiments over the last few years has been the San Francisco Biohub that we helped found and create to bring together researchers across UCSF Berkeley, Stanford, engineers, different folks to build different kinds of tools. And that model has worked really well for enabling collaboration. So we announced that we’re going to be creating a Biohub network in order to stand up a number of new Biohubs and have them all collaborate on building different kinds of tools.
And then the third area is, given the importance of understanding intelligence and how the brain works, neurodegeneration is a very important area of disease to understand. And also how important artificial intelligence is as a tool for accelerating science. We also are starting an institute called the Kempner Institute at Harvard to study natural and artificial intelligence. To really understand what the basis of intelligence is by bringing together folks who are more theoretical, focused on computer science or math with folks who are focused more on neuroscience, or cognitive science, or statistics to work together on that.
So a bunch of stuff to dive into here, and I think we’re quite excited about all of that. So I guess to kick this off for Ed. I’m curious when we talk about this idea of measuring and observing human biology and building tools around that, I’m curious what scientific questions you think are most important for us to be focused on here?
Ed: Well, I think we’ve all heard about the genome, the cells of our body contain about 30,000 or so different genes. Those genes encode for millions of kinds of molecules, biomolecules, and we have thousands of kinds of cells in the body. Actually, we don’t have a full list of all of them. I think why measuring and seeing is so important Mark, is because if you can’t see something, you can’t fix it. We can create vaccines, we can create antivirals, we can create antibiotics to fight the foreign invaders that enter our body, infectious diseases. But a lot of what’s going on in the body, whether it’s cancer or brain diseases, it’s our body fighting ourselves. We have to see what’s going on.
Priscilla: Yeah. And I think in reviewing your work, we talked a little bit about how this work is allowing people to answer new questions, look at blindness and Alzheimer’s, but what questions, what breakthroughs can you imagine being possible by focusing on measurement and imaging?
Ed: Well, in my home territory, the brain, we can’t explain things like thoughts and feelings, right? We don’t know how we take the external world, process it and make decisions. So there’s all these deep questions that are at the fundamental aspects of humanity, which are unanswerable right now. And knowing the answer to those questions, I think would really help us understand what it means to be human. And then there’s so many diseases like Alzheimer’s and blindness, and Parkinson’s where there is no cure, and frankly, treatments are few and far between. And the problem is that so many different things happen in the body during one of these diseases.
I think one of the key scientific questions to ask is can we figure out which change is the most important one? So we can go after that. If I drop a cup on the floor, the glass shatters. If I sweep up the glass, I’ve cleaned up the mess, but the initial problem is me dropping the cup on the floor. And if I keep dropping more and more cups on the floor, I’m just going to have to keep sweeping up the glass. I haven’t fixed the initial problem; I’ve been cleaning up a downstream problem rather than getting to the root cause.
Priscilla: Yeah. I mean, that’s the part where I think it’s so interesting to be able to see things live, instead of seeing a picture. That’s where moving from pictures to videos is going to be so fascinating. Also, understanding the human condition—that’s mind boggling.
Mark: So Ed, one of the things that I’m really curious about is you’re a real leader in the field around imaging and you pioneered this technology to basically blow up in the sense of massively expanding the volume of tissue, so that way you could take small tissues, right? Everything from the brain and make it a thousand times bigger, so that way people can see it with the naked eye and you can understand what’s going on more. Although, of course, that’s not in the living organism. I’m curious to hear you talk through what the challenges are, just because I think we probably have a lot of people on the line who are not imaging experts. So just to walk through some of the basic areas that need to get resolved or are bottlenecks today that are preventing high-resolution imaging from happening inside living organisms.
Ed: Yeah, that’s a great question. Imaging biology is really hard because there are different scales that you have to take into consideration. So if you try to understand how a cancer spreads in the body, or if you want to understand how the brain is generating a thought or is going wrong in a condition like Alzheimer’s, you’re dealing with large systems. And yet the building blocks of life are so tiny. They are a tiny, tiny fraction of a tiny, tiny fraction of the width of a human hair. We’re talking so microscopic that even the most powerful technologies out there are just beginning to be able to barely address the kinds of questions that we really are so desperately seeking answers to.
So the different scales that you have to deal with is so daunting, and that leads to all sorts of downstream problems. So for example, if I want to image something in the body, I have to get some energy into the body or measure energy coming out of the body, let’s say, but how do you aim that energy? How do you get it through bone? How do you get it deep inside the body where it needs to be without the signal being corrupted? So if I shine a light in my hand, like a flashlight through my hand, of course, the light gets blurred and you can’t see the nice ring of light through my hand. Something similar happens in microscopy where light will scatter or be absorbed by things like blood and bone and tissue.
So there’s a lot of physical limits of imaging that are very difficult to overcome. And then there’s the chemical side as well. Biomolecules are all made of the same alphabet, but in different orders. We have Carbon and Nitrogen and Hydrogen, and these atoms are shuffled around in different sequences, and so it’s very hard to tell things apart. If you think about the 30,000 or so genes in the human genome, which can code for millions of different molecules, I wish we could see a million different colors at the same time, but we just can’t do that. That’s so difficult to imagine how to do that, and so that’s one of the big challenges for the future of imaging.
Mark: Yeah. So when you’re talking about different kinds of energy and putting them into bodies, I mean, that’s how we end up with things like ultrasound, right? To do certain kinds of imaging instead of light, because light can’t always pass through tissues and using infrared because it passes through more and stuff like that. One of the areas that I’m really interested in is how you can connect some of these more physical approaches to microscopy that have existed traditionally with newer aspects of machine learning or artificial intelligence and labeling to be able to produce clearer images than what might be possible to observe otherwise. I’m curious what you see on the horizon there that you’re excited about.
Ed: Yeah, this is a very exciting area. And the intersection between chemistry, physics and artificial intelligence, I think is going to be one of the great productive collisions between scientific fields in the near future. I’ll just give you one example, Mark you alluded to this technology we developed that we call expansion microscopy. So we can take a biopsy from an actual human patient, we infuse it with a chemical that’s a lot like the stuff in baby diapers, add water, and we can swell it and make it a hundred or a thousand or even more times bigger. So it lets you see the fine detail that might happen, for example, early in a disease. Early in a disease it’s hard to diagnose it because the changes are literally very small. And if we can make those literally small changes big and obvious, that’s powerful, but there’s a price, right?
Now you’re getting a hundred times more data, you’re dealing with, if you have more colors, incredible dimensionality of complexity of the signals that emerge. And so a couple years ago, we actually showed collaborating with some doctors, that we could take cancer biopsies and expand them to reveal the very fine structure. And then we can use machine learning to classify those expanded biopsies. And we showed that we could actually improve the ability of doctors to assess disease. They could detect early changes in cancer better with the assistance of expansion and machine learning. And so that’s just the tip of the iceberg. I think the ability to get better data, not just more data, but better data, and then use machine learning to pull the signal from the noise, is just going to be one of the great synergies of the next several years.
Priscilla: So Ed, Mark and I have had the privilege of seeing your lab, and it’s amazing. And I think the thing is, what I realize is that it’s so unique and so far beyond what other scientists have access to. Tell us about how we can share this resource and share more broadly in the scientific community and what you expect these tools to be able to unlock if we manage to make it so that more people have the resources that you have.
Ed: Absolutely. Well, so making tools can require a lot of resources and a lot of destructive collisions between people from different backgrounds. You bring the computer scientists and the physicists together, you bring the chemists and the doctors together, you bring sometimes artists or designers into the mix. People like to talk about interdisciplinary science; I think we need omnidisciplinary science. And so one of the things that we try to do of course, is to always share as freely as possible, all the outcomes of our work. So when we make a technology like expansion microscopy that we were just talking about, or optogenetics, which as you introduced Priscilla, is the way that we control brain cells with light, we always try to give away that technology as freely as possible.
And one of the key things that is important to note is that these technologies, while they may be hard to generate in the first place, they’re really inexpensive to share and easy to use. And we really try to focus on education and teaching as well. So we, pre-COVID-19, used the whole of all sorts of workshops where people could come and learn how to use these tools and then take it home with them back to their institution or university. And so I think teaching and sharing are really at the core of taking these inexpensive and powerful tools and making it available to everybody because in the end, biology and medicine involve a lot of luck. And that means we have to share the tools with everybody because you don’t know necessarily where the next big discovery is going to come from.
Mark: So one of the things that I’m really curious about, and I guess this could be a question for either of you Priscilla or Ed. I mean, this doesn’t need to just be us asking Ed questions the whole time, but it’s one of the things that I think is really interesting if you’re trying to accelerate the pace of science, is trying to get a sense of where some of these discoveries or breakthroughs come from, and enabling more serendipity around that, right? Because right now there’s tens of billions of dollars in funding goes into science in the US alone, but even within that, there are still some big areas like tools development that we think could have a lot more funding.
There are areas like supporting younger scientists who are earlier in their careers where giving them more creative flexibility that we think can unlock a lot of ideas as well. And that’s a lot of what we’ve tried to experiment with at CZI so far. So I guess I’m curious for Priscilla, the things that we’ve done over the last five years and the different collaborative models, what has surprised you about where innovation has come from? And Ed, you run one of the largest labs at MIT, if not the largest, and you work with a lot of scientists who are early in their careers, who have gone on to do pretty awesome stuff as well. And I’m curious what you’ve seen in terms of patterns of where things come from, how you’d think about tools development. And if you were trying to accelerate the overall pace of science where you’d focus.
Priscilla: I would say that the serendipity part is where we’ve seen really exciting work. It’s actually just bringing together that folks that normally don’t get to work together. And Ed just listed off a whole range of folks, including artists, but what we’ve seen is bringing scientists, for instance, in our neurodegeneration network, we wanted to work on neurodegeneration, but bring together folks that have different perspectives. So we had folks that traditionally studied neuroscience, but we also brought folks who have spent their careers in other parts studying the immune system, studying the gut, studying human behavior. We brought together physicians who may see neurodegenerative diseases differently from the clinic.
And I think most powerfully, we brought patients into the fold. Patients were able to highlight what problems actually really mattered to them on a day-to-day basis. Patients got motivated and organized registries, brought others into the research process to accelerate work. And so when you just bring together, broaden the network of different backgrounds, different expertises, different lived experiences, you start asking different questions and you find different solutions. And that has been really exciting. And in our funding, we funded certain projects, but all sorts of things actually happened no one proposed, no one had known going in was going to happen.
And we’ve seen people building organoids, or brain chips, or different ways of actually seeing through imaging and through single-cell work that we didn’t expect. But Ed, you live this on a daily basis in your lab—maybe say something about what that looks like for you.
Ed: Sure. Yeah. I’d love to give a bit of a perspective and then hear maybe how these things might play roles in CZI and in the future as well. You mentioned lived experiences, Priscilla, and one of the things of course, that I think we are working hard now in science, is to really make things like inclusion and diversity, not added on things, but really core to the mission. And our group, one of the things that we’ve been really trying to do is to not just be inclusive, which might sound like the bare minimum, but to celebrate identities and different pasts and backgrounds that people take.
And it’s so important because again, I think when you’re dealing with something as complex as biology and medicine, but also as human as biology and medicine, the personal perspective I think is really important. And the kinds of viewpoints and backgrounds and scientific trainings and random things people might know from a different part of their life can have huge impact in creativity. Because in the end it is about creativity and helping collaborators work together. So I’m actually very curious at CZI, you mentioned how you saw these unexpected outcomes. Are their ways to be more deliberate, I guess, and to scale up the collaborative nature to get people working together across institutions or across disciplines more? I wonder.
Priscilla: I’ll chime in a little bit about the way that we think about collaboration within our networks, and then maybe Mark can say some words about the Biohub. For us, we really do prioritize the creativity piece. I think that’s the part that may surprise a lot of people not working in science on a daily basis. There’s not a formula, there’s not a clear route and it’s a lot about seeing a problem differently and being able to try different things and apply different techniques. It’s a recipe that doesn’t exist yet, but what we’ve seen is that we have to find smart people and our job is to give them great tools.
And we build a lot of those tools, but what we do is we put them freely in the hands of folks in the lab every day and allow them the freedom to do the work. We try not to be prescriptive, but we do put emphasis on making sure that folks both are connecting with others in our groups, but also making it easy for them to connect with others. And so I just spoke at a convening this morning in our Rare As One cohort where we try to create opportunities for folks to naturally connect with each other.
Ed: That’s wonderful. Yeah, catalyzing serendipity is really the key and that’s wonderful.
Mark: Yeah. I mean, I think my take on this from the last five years at the Chan Zuckerberg Initiative and the different science programs is that the closer that you can just physically get people working together, the better it seems to work and we’ve tried a range of different projects. Everything from, on the one hand just building tools as a set of engineers that we then give to the scientific community to use. And in general, that hasn’t worked quite as well as co-developing things with the scientific community. And then we’ve had a number of collaborations ranging from ones where we helped build different technology and then tried to coordinate with a number of folks in the scientific community.
One of the reasons why we’re so excited about the Biohub model is that it breached down barriers between universities. I mean, people who are researchers or engineers between Stanford and UCSF, you think okay, if they wanted to work together, it shouldn’t be that hard, but there hadn’t really been a lot of mechanisms or encouragement to do that before. And I just think that bringing some of that together has been really powerful in a way that seems so simple. So it’s in a lot of ways surprising that it’s been able to have as positive of an impact, but maybe we shouldn’t be that surprised about it.
I mean, I see in the different work that I do that sometimes if you have two different teams that you want to get to work together, literally just having them sit together in the same basic part of the office can make a big deal for increasing collaboration. So it makes sense that if you have different sets of scientists or engineers, and you want to get things to be more multidisciplinary, that just getting people to work together more closely is going to help out there. So sometimes some of this stuff is simple, but it just hasn’t been as emphasized in the past, and I’m glad that we can push on this. And it’s cool to see the impact that it can have.
Maybe to take the conversation in a somewhat different direction, and we’ve talked about imaging, we’ve talked about some of the ideas around where you can look for innovation and different things that have worked in terms of different collaborations. One of the other areas that we’re going to be investing a lot in over the next 10 years is this Biohub network. So the idea that we started with a single Biohub at Stanford, UCSF in Berkeley, and a number of really great scientists and technologists there are building tools. And the goal for the next 10 years on this is going to be just like in imaging microscopy, we want to take long-term efforts to build tools, to observe and measure important aspects of human biology.
But we haven’t identified yet what those tools should be that we want to build. And we have a bunch of ideas around this, but we also want to see proposals from the community and from different universities around what they should be. And one of the things that I’m curious to talk about and get both of your perspective on are, what kinds of tools you each think are really important that are good grand challenges that maybe we should be working on over the next 10 years to potentially build Biohubs around? Ed, I don’t know if you want to jump in there. And Priscilla, I think we’ve spent a little more time talking about this, but I’m curious to hear your answer to that one, too.
Ed: Yeah. Maybe I can throw out one idea. We’ve talked a lot about imaging and how important it is and how difficult it is, but the flip side, controlling biological systems, is also very difficult. And so with optogenetics, we got lucky; the natural world had made these molecules from bacteria and algae, that we could pop into brain cells and control them with light. I mean, a lot of that was serendipity, but there are literally thousands of other pathways in the body molecule cell types. How do we control all of them? If we could perturb or change a specific signal and turn off the ability of a cancer to spread, for example, that would just be amazing. So I think the flip side of imaging is control and it would be interesting to think a lot about how we could repair systems in a very focused and targeted way. Priscilla, do you have an area you think is interesting?
Priscilla: One, I’m really excited about getting other folks’ ideas because the Biohub networks, it’s a challenge to the scientific community because we’ll be accepting requests for applications. We will be putting out a request for applications soon. From different communities, say what do you think is possible if we gave you the freedom to build and work on a project for 10 to 15 years? What can you achieve? So that’s going to be really exciting to see and call to action. But if I’m going to put my nickel down on a few that I’m excited about, the immune system is super interesting. The immune system is like little signals and little doers that go across your body and both help us heal from when we’re sick and actually can also attack different cells for better or for worse.
When the immune system attacks yourself, that’s bad, but can we get it to attack cancer cells, to clean up cells that are sick or dying in our body? I think that’s super interesting. I think that our ability to actually understand in more real time how the body is expressing its various states, how do we know early on when an organ is sick? How do we know early on when an organ is showing signs of distress? Or how do we intervene at a minute level? That’s where the immune system comes in again, but we’re going to need the ability. That’s for time, the idea of dynamic precision comes into play because right now we get really, really rudimentary snapshots over time. But how can we get more accurate knowing when something changes in the human body?
That’s really interesting. How do we get more specific? Ed does incredible work in getting really deep into the brain, but how do we actually get precise about where an issue is? And how, where in a chemical pathway, something goes wrong? All of that is really exciting. And Steve Quake is going to be helping us issue that challenge to the broader scientific community. Give us what you got, what can you do if we could support you in building out a new Biohub to answer a question that’s critical to all of our lives?
Ed: So this new Biohub network, this could be anywhere?
Priscilla: Anywhere in the United States is what we’re ready for.
Ed: Very cool.
Mark: Yeah. Sorry, Priscilla. Keep going.
Priscilla: Oh, okay. I think what we’re looking for is actually just a place in the country where by setting up a Biohub to break down traditional barriers, how can we unlock that additional potential? Anywhere in the country.
Ed: That’s great. Yeah. I mean, the best ideas can come from anywhere and helping connect the dots I think, between people and disciplines is so key. So yeah, that’s wonderful.
Mark: All right. So, I mean, I’m curious just to go back to the last question a little bit. I mean, Ed, you were talking about control mechanisms and I mean, some of the work that you’ve done around optogenetics I think is just incredibly interesting. And probably most people listening to this haven’t heard much about that. But just the quick summary, and you should correct me if I’m getting anything wrong here is, using light and encoding things in the genetic sequence to be able to get different tissues, to give a signal or do something when they basically have light hit them. So you’re essentially able to control a tissue with light. I’m curious, how are you thinking about extending that? And when you talk about different control mechanisms as an important set of tools for the next decade, what else do you have in mind there?
Ed: Yeah. So cells in our body can take in many inputs. They can receive hormones, they can receive small molecules, they can receive vitamins, they can receive energy containing molecules, and then they generate so many outputs. They move, they grow, they divide. In our brains they help encode memories, or they go wrong in disease states. So inside the cell is this roiling sea of information processing, and information’s being processed through chemical interactions and chemical modifications. So a cell is just an incredibly dense ecosystem filled with molecules that are all talking to each other. So I think control is important for at least two reasons. First of all, if you see a lot of stuff inside a cell, that’s extremely important, right?
Because if you don’t see it, then how can you even begin to know what’s there and understand it? And suppose you see 10 different signals going off in a cell, as for example, a cancer cell starts to move, or as a brain cell starts to die. How do you know which of the 10 is the most important? Well, what you might want to do is to delete each one and see if it was actually important. Okay, we took that one out. Whoops, that was optional. Took a second one out, that didn’t change very much. Oh, the third one, now the system has changed fundamentally. Maybe that’s the important one. So it goes to this idea of causality. If you can perturb it, you can try to see what is the most important thing that contributes to a process.
But recently it’s also been fun to see that some of these tools are starting to be used in the quest to treat human diseases. So seven years ago, our group reported a molecule that converts red light into electrical signals. And it’s down from a specific species of algae, of all things. And we have used it as a tool for science and given it to hundreds of neuroscience groups to perturb brain circuits and try to see if we can understand how to shut down a seizure and so on and so forth. But in May, a group in Europe actually took the molecule and delivered the gene that encodes for the molecule into the eye of a blind man and he was able to start having partial vision again.
He was able to see lines of a crosswalk, he could make out household objects, like a box of staples if I recall, or a cup. And so this is very exciting, that control has this second life perhaps, of helping us remedy disease. If you could precisely control something, maybe you can also minimize side effects, because you’re not going to be controlling things that you don’t want to. So I hope that there’s going to be a lot of excitement about inventing or discovering ways to control these pathways in hopes that we can really understand them at a deep level, but also because maybe the new treatments of the 21st century are going to be about precision control.
Vaccines and antibiotics and antiviral drugs; part of why they’re so amazing is because they target exactly the thing that you’re trying to get rid of, right? And so that way you can maximize efficacy and minimize side effects. But when we’re talking about our own body, as it changes in cancer or Alzheimer’s, then it’s more subtle because you want to control one part of the body, but not other parts.
Mark: Yeah, that’s super interesting. I mean you just coasted by the result that some scientists have made it so that someone who is blind can now see a little bit, but that’s pretty amazing as a result. And I do think you get the sense that this precision control is a lot earlier and closer to the beginning here. So I think you make a very compelling case that that’s a very important set of tools to be focused on.
Ed: Yeah. Well it’s also just the beginning because every time a tool enters the world, people make new discoveries and we find out, you know what? What we thought was right, was wrong because science is about probing the unknown. And so that then feeds back and helps us figure out what tools to make next. It’s this wonderful virtuous cycle or positive feedback loop where each unknown that is found provides another seed for serendipity. And a lot of the great inventions are discoveries, right? CRISPR was discovered, PCR was based upon a molecule from Yellowstone Hot Springs, a microbe that lives in very hot water. Penicillin and aspirin were discovered from the natural world. The list goes on and on, how a lot of these so-called inventions really arose from a serendipitous discovery about the natural world.
Priscilla: So I want to spend maybe another minute on talking about tools and AI and machine learning. First of all, I think those are big buzz words that we hear a lot and actually do touch our lives in many ways. But I think one, if we experience the power of technology, being able to order dinner from my phone, I think it’s sometimes hard to understand how software, technology, AI, machine learning actually comes to life in the lab and what that actually unlocks and how we can use that to advance medical science. Because as someone who bridges these worlds, I think it’s an interesting divide where what’s possible in the consumer world is not yet possible or looks really different in the scientific and medical world. So can you walk us through some examples of what machine learning or artificial intelligence actually looks like and unlocks for us in science?
Ed: Yeah, sure. Maybe I can give a couple examples, but then I’d also love to hear what you all are planning at CZI and in the Biohub network. And a lot of these new system-level designs are bringing forth to revolutionize how science is structured and how tools are developed, both topics dear to my heart. I’ll give one example. So the brain is really complicated, right? So if you take a cubic millimeter, the size of a grain of sand of your brain, that contains 100,000 brain cells with a billion connections between them, which are known as synapses. And so tracing all of that by hand would take forever, and so one of the exciting areas that AI and machine learning has made leaps and bounds in recent years is in biomedical image analysis.
You can take an image which has incredible detail, and then you can have that machine learning algorithm examine how a human does it. And over time it can start to replicate what the person does. So if you take this piece of brain tissue and you want to make a map of it, then you have all this image data, and then machine learning can start to trace the wires of the brain. So that’s going to have, I think, a lot of real impact in the coming years. In the short term, knowing how the brain changes in a certain brain condition like epilepsy or Parkinson’s will help us understand how information flow, information processing in the brain is altered.
I think right now we can tell whether a brain cell is alive or dead, or we can look at its gene expression, but we don’t have great ways of examining how the wiring, the way that information flows, is altered. And machine learning is really one of the key tools that’s helping deal with the enormous amounts of image data that comes out. I mean, nobody wants to sit there tracing a billion things per cubic millimeter by hand. And so machine learning is really a way to automate that and to fix errors in the trace thing and to make it better. I mean, another interesting, and so far, maybe partly hypothetical impact of this, is that if you have really good maps of the brain, then feedback can help make better kinds of AI.
I think there’s a lot of desire to create AIs that have ethics, that are creative, that recapitulate the best parts of what it means to be human. And knowing how the brain does that information transfer and flow and transformation might be one way to galvanize AI in an interesting direction. Another example, I think that’s really cool is just how much genetic data and gene expression data is being generated right now. So you have this genome in every cell of your body, each gene can be on or off. I mean, it’s a bit more complicated than that, because you could have analog gradations. And so dealing with 30,000 genes being on or off or some more nuanced gradation, how do you deal with that sheer amount of data?
So fighting patterns of the data. Oh, that’s a cluster of cells over there. Oh, that turns out to be a cancer cell. Oh, that’s a cluster of cells over there. That’s the immune cell. And you can start to look at how they relate to each other. So I think there’s a lot of just sheer quantity of data, but also looking for patterns in space and across time. Anyway, I’d love to hear how you all are thinking about it as you launch these new initiatives as well.
Mark: Well, one point that you talked about around understanding how biological and natural intelligence work can be important for some of the new innovations and artificial intelligence. I mean, that’s a big theme of the Kempner Institute that we’re helping to create at Harvard. And a lot of the idea there is these same principles around bringing together different disciplines, in this case, it’s neuroscience which Harvard has a great program on, cognitive science, statistics, math, theoretical computer science, as well as engineering to build these tools. And the idea there is that you have new AI tools that are helping us measure things about the human brain for example, all of the connections that you talked about.
At the same time, if we understand how the human brain works, that can probably create some inspirational advances for artificial intelligence, too. So the idea that you want to study both of those things at the same time by bringing neuroscientists and engineers and computer scientists together, I think is a big part of the theme that we’re deliberately pushing on here. And to be clear, AI work and a lot of the advances haven’t all come from biologically inspired models. A lot of it has just been statistics and computer science, but I do think that there’s an important role here and that potentially some really important advances can be unlocked through understanding neuroscience and the structure of the brain more. So I’m quite optimistic about that.
In terms of making some fundamental advances in AI, across the rest of everything that we do, I tend to have this view of AI that it’s not a singular product. I think sometimes people think about these embodiments, whether it’s a robot or an assistant or something like that, but that’s not really how AI shows up in our lives. It’s just a tool that makes increasingly everything that we have, better and a little more personalized or adaptive or efficient. So that will continue in everything from the imaging work that we do to sequencing work, and identifying infectious diseases, and just helping people search for information in the scientific community more easily. Just all these things that we do to improve.
One of the things though is that a lot of the AI techniques today are somewhat intensive. And they require engineering and they require computational tools that historically a lot of labs, it’s been somewhat of a constraint on how far they can push that. So a lot of what we’re trying to do with these bigger centers and the Biohub network is basically have more resources around technology, both people, engineers and computational resources to be able to do more of that work. But it’s certainly an area that we’re quite excited about. All right. So we’ve been going for a while. So it may make sense to wrap up soon, but I wonder.
One closing question here for everyone, could be in addition to everything that we’ve talked about today, what are you most optimistic about for the future? And in terms of the scientific advances that will get made over the next five to 10 years? Maybe there can be some new themes apart from what we’ve talked about so far, but if you want to double down on stuff that we’ve talked about so far, then that’s cool, too.
Ed: Well, one thought that we haven’t talked about yet is this idea of ground truth, getting down to the fundamental building blocks. And if you look at other sciences, like chemistry, there was a period of time when it was really hard to make a chemical. And then chemistry matured and we have the periodic table, we know about chemical reactions, and now you can make Teflon and nylon and computer chips, and the list goes on and on. Chemistry became a science in a mature form, you can do it. And physics underwent a transformation, too. Previously physics was able to do certain things, but not so great at other things. And then there’s a period of time in the early 20th century, when quantum mechanics came along and people could suddenly start to build microchips, and lasers, and cell phones, and radar, and all sorts of stuff, electronics—physics matured.
I guess maybe the one thing I’m most excited right now is this idea if we can really understand the fundamental building blocks of life and how they all talk to each other and how they go wrong in disease states, maybe we can make this very high-risk, very difficult science of biomedicine into a mature science. And I think these tools that we’ve been talking about over the past hour or so are really at the core of what’s enabling that to happen, right? You can see the sequence and it’s just the beginning. I think that’s really going to revolutionize how biology and medicine as sciences operate in the years to come.
Mark: Yeah, I think that that’s going to be awesome. I’m curious when you say the basic building blocks, what you have in mind. I mean, this is an area that we’ve been really excited about, too, with a bunch of the projects that we’ve been engaged with around the Cell Atlas, the analogy of chemistry, I think is a good one because when you take an intro to chemistry class, one of the first things you learn about is the periodic table and our understanding of all the elements, which it’s at least at a certain level, the building blocks for all atoms beyond that. And we don’t really have that in biology yet.
There’s no catalog, or soon there will be, but to date there hasn’t really been a catalog of here are the 10,000 types of different cells that make up a human body. And here are the different states that they can be in, here’s how you can move them between states and things like that. But I’m curious if that’s what you have in mind or if that’s a piece of it, or if you think that it’s something that’s more fundamental and even more basic building blocks than that, that we really need to get at as well.
Ed: It’s a very important piece of it. And these human cell efforts, it’s embarrassing that we don’t have a list of all the cell types of the human body. What that means is if you have a drug that you’re trying to use to treat a disease, it might affect a cell you’ve never heard of in a way that you cannot predict from first principles. So I think there are two other levels that are really exciting that are coming into view. One is the level of molecules. How does a molecule that let’s say enters a cell, change what the cell does? And then when the cell starts to move around or divide or do its thing, that’s being expressed through molecules, let’s say on the cell surface that help that cell talk to its neighbor.
And molecules of course, are so tiny that until fairly recently, it’s been unimaginable to do molecular content imaging with molecular spatial precision at scale, but that’s now coming into view. And the other stream is the body itself. One of the big, I think, features of many medical problems is just how distributed they are. Priscilla mentioned the immune system earlier. Immune cells will wander throughout your whole body, collecting information, changing autoimmune diseases. Maybe some pathways have become overactive, different conditions of course, of underactive immune systems. And so how do you deal with some of these complexes in the entire body?
That’s another realm where understanding how the building blocks in this case, how a cell interacts with different components as it wanders around, would be really exciting to be able to see and perhaps control.
Priscilla: And for me, Mark, as a pediatrician, I just look forward to be in a world where we can actually give satisfying answers to patients. We’re getting better and medicine has continuously improved over the centuries, but it’s still rudimentary. Sometimes we give people these labels, then what? Autism is a giant bucket of things that are actually happening. You have a seizure, but what causes it? In adult things, you have high blood pressure, but what’s actually happening? And we give these big, overarching diagnoses without really satisfying explanations or clear effective treatments. Depression is another one I’ll put into this bucket. There’s so many different manifestations.
And to be able to get to that ground truth that Ed is talking about, and to be able to say this is what’s happening, this is the pathway, this is the molecule that’s not working right. And to be able to then intervene, that’s power. That’s the ability to actually use science to directly answer a patient’s question and help them actually get effective treatment quickly. That’s exciting. And I don’t think that’s out of reach for us given the tools that we have, our ability to analyze data, the ability to understand cells and what’s happening in a real-time basis. That would be a big deal, and I look forward to being able to answer those questions for patients within my lifetime.
Mark: All right. Well, I think we covered a lot of ground here and this was a really interesting discussion. Thanks Ed for joining us and thanks for everything that you do. I think that your work is some of the most inspiring work out there, and it’s really awesome to see all the advances that you in the lab and your collaborators make. And Priscilla, I know that we’re super excited about the next chapter for the Chan Zuckerberg Initiative and the science program. And I think we’ve learned a lot over the last five years about which different types of collaborations can work. We only talked about a handful of them today, but to your last point, Priscilla, some of the stuff where we’re empowering patients and doctors in addition to scientists and technologists, there’s all different kinds of collaborations.
And I think I’m really optimistic for the next five to 10 years that we’ve started to get a sense of where there’s more leverage in terms of bringing people together and what tools need to exist, and what collaborations are most effective. And it’s really inspiring getting to work with all the different folks at CZI and the partners that we work with and the folks at the Biohub. There have just been so many just incredibly talented and brilliant folks who we’ve met and gotten a chance to learn from along the way. So thank you again to Ed for joining us for this, and we’ll see you all soon.
Priscilla: Yeah. Check out our annual letter and we’ll be sharing the look back on our five years of science in our symposium. So if you want to learn more, those are two really great places.
Mark: Yeah. That’s a good call. The symposium that we did yesterday, I thought it was a great summary of all the work that we’re doing. So if you want to look at the Chan Zuckerberg Initiative website, it’s there if you want to check it out and go deeper on all this. All right. See you all soon.
Priscilla: Thanks, Ed.
Ed: Great talking to you. And really excited to see all the great science and to collaborate with you all and help advance this great mission.
Priscilla: Let’s do it.
Mark: Awesome.
Learn more about our work in science technology.
###
About the Chan Zuckerberg Initiative
The Chan Zuckerberg Initiative was founded in 2015 to help solve some of society’s toughest challenges — from eradicating disease and improving education, to addressing the needs of our local communities. Our mission is to build a more inclusive, just, and healthy future for everyone. For more information, please visit chanzuckerberg.com.