Enhancing Rigor and Reliability of Single-Cell Data Science
Project Summary
This project will develop software packages and graphical user interfaces to enhance the rigor and reliability of single-cell data analysis and tool benchmarking. The team will address the widespread issue of inflated false discovery rates (FDRs) in single-cell data analysis. Previously, this team has reported that several popular bioinformatics tools have unexpectedly large FDRs far exceeding the claimed FDR threshold due to the use of ill-posed p-values. Accordingly, the team developed the statistical method Clipper to implement p-value-free FDR control and avoid the statistical complications and computational burdens of obtaining well-calibrated p-values. This team will generalize and adapt Clipper to various single-cell analyses, including the detection of differentially expressed genes and the identification of CRISPR perturbation targets, to ensure valid FDR control.
The project will also account for the issue of data reuse, which complicates statistical inference and inflates FDR (e.g., identifying differentially expressed genes among cell clusters identified from the same data). The project will also develop a versatile simulator to generate realistic single-cell multi-omics data and spatial transcriptomics data with ground truths, thus allowing the single-cell community to perform fair and informative benchmarking of computational tools. The simulator will be comprehensively designed to include various cell states (discrete cell types and continuous cell trajectories), technologies (spatial and sequencing omics), experimental factors (cell number, library size, batch effects, and conditions), and data formats (sequencing reads and/or summarized count matrices).
Investigators
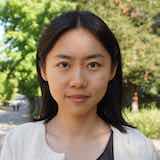