Machine Learning to Identify Soft Tissue Molecular Signatures
Project Summary
Pelvic organ prolapse, where a woman experiences a vaginal hernia and long-term pain, affects up to one third of women during their lifetimes. Ten percent of all women in the U.S. undergo surgical intervention for pelvic organ prolapse, yet this procedure fails 15 to 30 percent of the time due to mechanical incompatibilities and an insufficient understanding of the ever-changing soft tissue composition, which predisposes a woman to the condition. Therefore, these soft tissue molecular markers may serve as predictive indicators of individual prolapse risk and allow clinicians to identify women likely to benefit from therapeutic interventions at an earlier stage. Therefore, an urgent need exists for imaging methods that can relate soft tissue composition/mechanics with probability of pelvic organ prolapse development to improve care for women affected by this condition. Without the development of imaging tools to enable earlier detection and to facilitate targeted interventions, women will continue to suffer long-term effects, with many undergoing ineffective surgeries.
The project’s long-term goal is to establish a multimodal imaging platform assisted by machine learning algorithms to analyze soft tissue molecular composition for early pelvic organ prolapse diagnosis. The overall objective of this proposal is to establish feasibility of co-registering spectral photoacoustic (sPA) imaging and hyperspectral coherent Raman imaging (CRI), integrated through machine learning, to identify soft tissue molecular characteristics indicative of tissue that is more susceptible. This project’s central hypothesis is that, by combining data from both imaging modalities with quantitative metrics as ground truths, machine learning algorithms will be capable of identifying key signatures in sPA and CRI images, as well as soft tissue biomechanical parameters.
Investigators
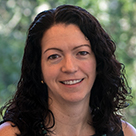
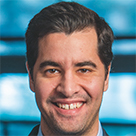
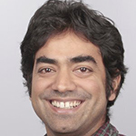